What is a chess engine’s raison d'être? I ask the question, only partially tongue in cheek, because of the early reaction to Part I of this review, which hit the web on Sunday. For those who have not read Part I, here’s a brief recap: Fritz 17 includes the new Fat Fritz engine, a neural network with roots in the open-source Leela Chess Zero project. After describing the difference between traditional engines and neural nets, I then discussed the results of my early testing of Fat Fritz, and in particular, its performance in six engine-engine matches. I found that Fat Fritz was marginally weaker than the strongest Leela nets and development versions of Stockfish on my machine, something that appears to be borne out by further independent testing. Almost immediately some Twitter followers began to ask (I assume in good faith!) why they should be “excited” about a commercial neural net engine when Leela and Stockfish, both free to download and use, are “better.” It’s a good question, but to answer it, we have to do a bit of back tracking and ask another one: what’s the point of using a chess engine at all? Let’s begin by admitting the obvious. Almost every mature, well-written engine is more than strong enough to defeat any human alive. It’s gotten to the point that the authors of Komodo are, in conjunction with Chess.com, sponsoring handicap matches between Komodo and masters, even spotting FIDE Masters a full knight. The war is over, folks. Our silicon friends have won. No one plays the engines anymore. They’re just too strong. But that immense, unfathomable strength is what makes them so important for human players to use and understand. Anyone with even a modern cellphone effectively has a Super-GM analyst and coach at their beck and call, willing and able to answer questions 24 hours a day. In 2008 I referred to Fritz, then the strongest engine around, as something akin to the spell-check function in your word processor, a utility to catch blunders during analysis. In hindsight, this was a mistake. By that point Fritz, Hiarcs, Rybka, and Shredder were, from a practical perspective, the strongest chess playing entities on the planet. What they lacked in “understanding” they more than made up for in tactical ingenuity, and already they were critical parts of top-level preparation. Kasparov’s 2005 victory over Rustam Kasimdzhanov was due, in no small part, to a key opening idea that Deep Junior had found in 2003.
[pgn] [Event "Linares"] [Site "?"] [Date "2005.??.??"] [Round "?"] [White "Kasimdzhanov, R."] [Black "Kasparov, G."] [Result "0-1"] [ECO "D48"] [WhiteElo "2678"] [BlackElo "2804"] [Annotator "Hartmann,John"] [PlyCount "72"] [EventDate "2005.??.??"] 1. d4 d5 2. c4 c6 3. Nc3 Nf6 4. e3 e6 5. Nf3 Nbd7 6. Bd3 dxc4 7. Bxc4 b5 8. Bd3 Bb7 9. O-O a6 10. e4 c5 11. d5 Qc7 12. dxe6 fxe6 13. Bc2 c4 14. Nd4 Nc5 15. Be3 e5 16. Nf3 Be7 17. Ng5 O-O $1 $146 {Kasparov had prepared the Meran with White for his 2003 match with Deep Junior, and in the midst of his preparation, he noticed that the computer would allow White to win the exchange in this position. In fact, Deep Junior would evaluate the position as favoring Black, despite the material deficit! This led Kasparov to more thoroughly investigate the idea, and he concluded that the computer had stumbled upon a rather remarkable positional sacrifice of material. While the position did not arise in the 2003 match, it did become part of Kasparov’s opening database. Kazimdzhanov was unaware of this, and played into Kasparov’s analysis.} { After} 18. Bxc5 Bxc5 19. Ne6 Qb6 20. Nxf8 Rxf8 {Kasparov had full compensation for the exchange and went on to win the game in fine style.} 21. Nd5 Bxd5 22. exd5 Bxf2+ 23. Kh1 e4 24. Qe2 e3 25. Rfd1 Qd6 26. a4 g6 27. axb5 axb5 28. g3 Nh5 29. Qg4 Bxg3 30. hxg3 Nxg3+ 31. Kg2 Rf2+ 32. Kh3 Nf5 33. Rh1 h5 34. Qxg6+ Qxg6 35. Rhg1 Qxg1 36. Rxg1+ Kf7 0-1 [/pgn]If the world’s elite were already “cyborgs” in 2008, their chess an amalgam of carbon- and silicon-bases intelligence, how indisputably truer this is today. The seeming omnipotence of the engines revolutionized opening preparation almost overnight, and players grew confident playing “ugly” but pragmatically valuable ideas.
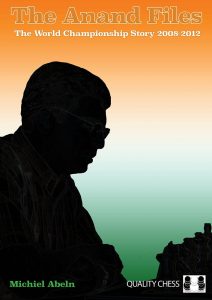
The team thought it was not Vishy’s memory that had deteriorated since the Bonn match. Instead it was clear that the amount of knowledge he needed to remember was growing exponentially. More and more lines were being analysed with strong engines that didn’t exist just a few years earlier. … Anand also felt the stress of needing to know all his lines perfectly because of Topalov’s feared new supercomputer. (§5.1, “On the Rebound”)
Today Stockfish is ubiquitous at all levels of chess. It is embedded into popular web interfaces, its evaluation taken as gospel. Players have internalized silicon insights such that the machines set the standards for truth and falsity. If Stockfish says it’s a good move, it’s a good move, and nothing more need be said. But after AlphaZero, that is beginning to change. What makes neural net based engines like AlphaZero, Leela, and Fat Fritz so valuable is that they bring a set of new ideas into chess, expanding our notion of the possible once more. Jokes about “AlphaZero moves” abound in post-game interviews and game annotations, and implicit in them is the recognition that a new source of information and inspiration has arrived. Where Stockfish returns 0.00 evaluations, neural nets tend to consider who has initiative, who has more ways to lose, and they factor that into their output. The world’s top players are already scrambing to mine NNs for their insights, and this is trickling down to less augustly rated players like me. Let’s be clear. If all you want is blunder-checking, there’s no need to go beyond Stockfish. But if you want to build understanding, if you want to generate your own ideas in conjunction with the engines, you need to use all the tools at your disposal, and you need to understand their strengths and weaknesses. Alpha-beta engines like Stockfish are fantastic at calculation, defense, and (with tablebase input) endgames, but they lack elite positional sense and they can underestimate initiative and “compensation.” Neural networks like Leela and Fat Fritz have brilliant understanding, but sometimes miss concrete continuations, and they can meander in winning endgames. Used together, taking the best from each, there is the possibility to generate fascinating ideas and insight into positions of all types. Like Stockfish, Leela is an open-source product, free to download and use. So why should someone pay $89.95 for Fritz 17 and Fat Fritz, especially if (as we saw in Part I) it is marginally weaker than both Stockfish and Leela? Bracketing everything else that comes in the Fritz 17 package – the new Ginkgo / Fritz 17 alpha-beta engine, the automated install of Leela, the updated GUI and GUI features, and the six months of Playchess.com membership – it seems to me that the question comes down to style and the generation of ideas. An engine’s objective strength is important, but the subjective element – what kind of ideas it generates – is equally, if not more, essential to me. Does Fat Fritz have a different style than does Leela? Does it “see” different possibilities in a position, and can it help us, the end-users, generate insights that we might not have otherwise? Subjective Style and Tests After a few weeks using two iterations of Fat Fritz, I would argue that (a) it does have a somewhat different style than Leela, and (b) that serious analysts will find it worth the purchase price. Fat Fritz is aggressive in a less alien way than an alpha-beta engine like Stockfish, valuing initiative and complexity. Its weaknesses are similar to those of Leela: it sometimes misses tactics, it can drift in endings, but its ‘understanding’ is very, very high. Compared to Leela, Fat Fritz feels stronger tactically (even if tests don’t entirely bear this out), more comfortable in messier positions, and perhaps less positionally oriented, but this is impressionistic. Both Fat Fritz and Leela are ridiculously strong, and while I can watch how the evals move in the engine box, it’s sometimes hard for me to judge what those evaluations mean. Knowing my limitations, I asked a titled friend who has looked at a lot of the publicly released games for his opinion. He confirmed that he too saw a stylistic difference between Fat Fritz and Leela, mentioning a few specific points.
- Fat Fritz loves outposts.
- Fat Fritz is better at pawn races / play on both wings.
- Leela likes domination and space.
- Leela has a better sense of material.
Ultimately readers will want to take a look at the 1046 downloadable Fat Fritz games that accompany this review to make their own assessments. (Thanks to ChessBase and CEGT for allowing me to include their test games in the downloadable file.) Some specific games stood out. Chess engines are not immune to blundering, especially at quick time controls, and both Stockfish and Fat Fritz played howlers that led to quick losses. These are games #160 and 550 in the downloadable pgn.
[pgn] [Event "ChessBase.com games 20m+10s"] [Site "DESKTOP-DPJ1O5S"] [Date "2019.10.26"] [Round "60"] [White "Stockfish 10 64 POPCNT"] [Black "Fat Fritz rtx"] [Result "1-0"] [ECO "C45"] [Annotator "Hartmann,John"] [PlyCount "73"] [EventDate "2019.??.??"] {#160} 1. e4 e5 2. Nf3 Nc6 3. d4 exd4 4. Nxd4 Nf6 5. Nxc6 bxc6 6. e5 Qe7 7. Qe2 Nd5 8. c4 Ba6 9. b3 g6 10. f4 d6 11. Qf2 Nf6 12. Be2 dxe5 13. O-O Ne4 14. Qe1 Qc5+ 15. Kh1 Qd4 16. Bf3 Qxa1 17. Qxe4 Bb7 18. Qc2 O-O-O $4 19. a3 Bxa3 20. Bxa3 Qd4 21. fxe5 Rhe8 22. Bb2 Qd7 23. Re1 Qf5 24. Be4 Qf4 25. Bc3 f5 26. exf6 c5 27. Nd2 Kb8 28. Qb1 Rxd2 29. Bxb7 Rc2 30. Rxe8+ Kxb7 31. Re1 Rxc3 32. Rf1 Qh6 33. f7 Qf8 34. Qe4+ c6 35. Qe8 Qd6 36. f8=Q Rc1 37. Qa8+ 1-0 [/pgn]
[pgn] [Event "3m+2s LR 1.4"] [Site "FF fp16 vs SF 8core"] [Date "2019.11.10"] [Round "68"] [White "Fat Fritz"] [Black "Stockfish 061119 64 POPCNT"] [Result "1-0"] [ECO "B97"] [Annotator "Hartmann,John"] [PlyCount "47"] {#550} 1. e4 c5 2. Nf3 d6 3. d4 cxd4 4. Nxd4 Nf6 5. Nc3 a6 6. Bg5 e6 7. f4 Qb6 8. Nb3 Nbd7 9. Qf3 Be7 10. O-O-O Qc7 11. Bd3 b5 12. Bxf6 Nxf6 13. g4 b4 14. Ne2 a5 15. g5 Nd7 16. Kb1 a4 17. Nbd4 a3 18. b3 Ra5 19. Rhf1 O-O 20. e5 Nc5 $4 21. Bxh7+ Kxh7 22. Qh5+ Kg8 23. Rf3 g6 24. Qh6 1-0 [/pgn]King’s Indian structures are notoriously hard for traditional alpha-beta engines to understand. Fat Fritz, perhaps because it was fed games with closed positions during training, appears to do well in them. Here are two games (#304, 834) I came across where Fat Fritz uses the standard …g4-g3 pawn break / sac to attack the White king.
[pgn] [Event "2m+1s RTX 2060"] [Site "Match #2"] [Date "2019.11.04"] [Round "4"] [White "Lc0 v0.22.0, T40B.4-160."] [Black "Fat Fritz"] [Result "1/2-1/2"] [ECO "E99"] [Annotator "Hartmann,John"] [PlyCount "81"] {#304} 1. d4 Nf6 2. c4 g6 3. Nc3 Bg7 4. e4 d6 5. Nf3 O-O 6. Be2 e5 7. O-O Nc6 8. d5 Ne7 9. Ne1 Nd7 10. Be3 f5 11. f3 f4 12. Bf2 g5 13. b4 Nf6 14. a4 h5 15. a5 g4 16. c5 g3 $1 17. hxg3 fxg3 18. Bxg3 Ng6 19. a6 b6 20. Bb5 bxc5 21. Bc6 h4 22. Bf2 Rb8 23. Nb5 Rb6 24. bxc5 Rxa6 25. Nxa7 Rxa1 26. Qxa1 Nh5 27. Nxc8 Qxc8 28. Be3 Qd8 29. Kh2 Ngf4 30. Rf2 Ng3 31. Bb5 Qg5 32. Qc1 Rb8 33. Bd7 Nge2 34. Qd1 Qg3+ 35. Kh1 Rb2 36. cxd6 cxd6 37. Be6+ Kh7 38. Bf5+ Kg8 39. Be6+ Kh7 40. Bf5+ Kg8 41. Be6+ 1/2-1/2 [/pgn]
[pgn] [Event "CEGT 40_20"] [Site "Werner"] [Date "2019.11.13"] [Round "52"] [White "Stockfish 10.0 x64 1CPU"] [Black "Fat Fritz 1.0 (in lc0) Cuda"] [Result "0-1"] [ECO "E92"] [Annotator "Hartmann,John"] [PlyCount "95"] [EventDate "2019.??.??"] [SourceTitle "CEGT 191117 update"] [SourceDate "2019.11.17"] [SourceVersion "1"] [SourceVersionDate "2019.11.17"] [SourceQuality "1"] {#834} 1. d4 Nf6 2. c4 g6 3. Nc3 Bg7 4. e4 O-O 5. Be2 d6 6. Nf3 e5 7. d5 a5 8. Bd3 Nfd7 9. a3 f5 10. O-O f4 11. Be2 g5 12. Nd2 Nf6 13. f3 Nbd7 14. b3 h5 15. Bb2 Rf7 16. b4 Nf8 17. c5 g4 18. Nc4 g3 19. cxd6 cxd6 20. bxa5 N6h7 21. Bd3 Ng6 22. Qd2 Ra6 23. hxg3 fxg3 24. Ne2 Nf4 25. Nxf4 Qh4 26. Nh3 Bxh3 27. gxh3 Ng5 28. Qxg5 Qxg5 29. Bc3 Ra8 30. Rae1 Rc7 31. Bb4 Rac8 32. a6 bxa6 33. Ne3 Rc1 34. Kg2 Rxe1 35. Rxe1 Rc1 36. Re2 Qd8 37. Bd2 Ra1 38. Nc2 Rb1 39. Ne3 Rb3 40. Bc4 Rxa3 41. Nc2 Ra4 42. Bb3 Ra5 43. Bxa5 Qxa5 44. Bc4 Bh6 45. f4 Bxf4 46. Ne1 Qb6 47. Nf3 a5 48. Ne1 0-1 [/pgn]Stockfish remains a vastly strong competitor, and in more than a few cases it simply outcalculated Fat Fritz. Check out this (#607) demolition job.
[pgn] [Event "7m+3s LR 1.4"] [Site "Ryzen 1700 8core RTX 2060"] [Date "2019.11.16"] [Round "5"] [White "Stockfish 141119 64 POPCNT"] [Black "Fat Fritz"] [Result "1-0"] [ECO "E16"] [Annotator "Hartmann,John"] [PlyCount "71"] {#607} 1. d4 Nf6 2. c4 e6 3. Nf3 b6 4. g3 Bb7 5. Bg2 Bb4+ 6. Bd2 Be7 7. Nc3 O-O 8. Qc2 Na6 9. a3 c5 10. d5 exd5 11. Ng5 Nc7 12. Nxd5 Ncxd5 13. cxd5 Re8 14. O-O-O b5 15. h4 b4 16. e4 Bf8 17. f4 d6 18. Rde1 bxa3 19. Bc3 axb2+ 20. Kb1 g6 21. h5 Bg7 22. Nxh7 Nxh5 23. g4 Nxf4 24. Ref1 Nxg2 25. Nf6+ Kf8 26. Rh7 Re5 27. Bxe5 Ne3 28. Nd7+ Ke8 29. Qf2 Nxf1 30. Rxg7 Nd2+ 31. Kxb2 Nc4+ 32. Kb3 Qe7 33. Nf6+ Kd8 34. Rg8+ Qf8 35. Rxf8+ Ke7 36. Rxa8 1-0 [/pgn]Readers are encouraged to point out and discuss other interesting games and positions in the comments to this post. What about specific analytical examples? I mention one in the December issue of Chess Life, where Fat Fritz comes up with a new idea in Fischer-Geller (Monaco, 1967). Here are a few more more esoteric positions I tested using:
- Fat Fritz, running on a RTX 2060.
- Leela, running the TCEC network T40B.4-160 remotely on a RTX 2080.
- the then-current development version of Stockfish (SF dev), running on four cores of a Ryzen 7 1700 at 3.7Ghz.
All six positions (with accompanying analysis) are provided in a pgn file at the end of this article, along with a few bonus positions / engine analyses that were cut for space.
1. Gusev-Averbakh
Here Gusev famously sacrifices his queen with 24. Qxe5!!, ending up in a position where White has rook and two bishops for two rooks and the queen, but is absolutely dominating. SF dev does not see the correct move in any reasonable timeframe – the last I saw, it may get there after about 70 ply! – but Fat Fritz wanted to sac the queen in all of 11 seconds. Leela (running the TCEC network T40B.4-160 on a RTX 2080) took a bit longer, but after 44 seconds, it came around.
2. Svidler-Grischuk
One of the most heavily analyzed positions of recent years comes from the 2013 game between Svidler and Grischuk at the London Candidates. Grischuk famously sacrificed a piece after 12. f4 Nxc4 and the board exploded in complications. All three engines wanted to avoid Grischuk’s idea with 12. Bg5, echoing Kotronias’ analysis in his book for Quality Chess. After 12. f4, they unanimously agreed with Grischuk and wanted to pitch the piece.
3. Minski and Afek, Benko 90 JT, 2019
One of the relative weaknesses of NN engines is that they are not as precise in deep calculations, especially in the endgame. Studies are an interesting place to test this, and here, in a study by Martin Minski and Yochanan Afek, they miss the mark. SF dev sees the correct idea (1. a7!) almost immediately, but both Fat Fritz and Leela get stuck on 1. Bd4+ Kd5 2. Rd1 Ra2 3. Bg1+ Ke4 4. a7 Nf5, when there is no win. The solution is provided in the pgn file of examples at the end of this article.
4. Van Breukelen, Schakend Nederland, 1990
Just for fun, here’s a study that no engine (that I know of) can answer from the initial position. Leela is the first to see the correct answer after being fed 1. Nf6+ Bg7, while SF dev and Fat Fritz only understand what’s going on after seeing 3… Kxh5. What’s interesting is that after analyzing Black’s third move and going back to the initial position, Stockfish ‘gets’ it, while the neural net engines ‘forget’ what they have just analyzed. It appears that Fat Fritz and Leela cannot access their stored hash tables when moving backwards in a game, which may hamper them in analysis mode. An aside: another difference, this time in favor of the NN engines, is that running Fat Fritz or Leela in multi-PV mode (showing two more lines in the engine window) does not have any cost, whereas Stockfish is weakened slightly in multi-PV mode. Check out the pgn file at the end of this article for the complete solution.
5. Botvinnik-Dvoretsky
Engines are forcing us to revisit and revise much of what we know about chess. Sometimes they refute previous analysis, but sometimes they simply remind us that what is possible on a chessboard is often much broader a category than we have imagined.
Mark Dvoretsky writes in School of Chess Excellence 3: Strategic Play of this position, taken from a game he played in a 1964 simultaneous exhibition against Mikhail Botvinnik. He writes: “After playing the opening excellently, White then acted uncertainly and ended up in an inferior position. The move in the game (23. … f6) seemed to me to be completely natural – it attacks the enemy centre, and includes the inactive dark-squared bishop in the play.” (84) Vladimir Simagin was shown the game and asked the young Dvoretsky, “Why weaken the position of your king and give White counterplay? Surely you can manage without this move? … Look at the ‘holes’ in the opponent’s position on the light squares. Your knight is dreaming of reaching there.” (ibid.) And only then did Dvoretsky consider 23. … a5!! (his exclams), which will drop the knight on b4 and reroute it to c2, d3, or d5. I was interested to see how different types of silicon intelligence would handle this position. All three engines think that there are multiple decent moves here for Black, including 1. … a5 and 1. … Qd7, with scores that are nearly identical. 1. … f6 is also seen as entirely viable, with all engines scoring it around 0.00. 1. … a5 may be the best ‘human’ move, but one of the great lessons of our ‘metal friends’ is the expansion of moves that humans have to consider. Conclusions In the final analysis, I think Fat Fritz is an important new tool for players and analysts, a terribly strong network / engine with a novel and interesting playing style. Chess players have grown spoiled by the availability of Leela and Stockfish, both open-source and free to use, but Fat Fritz represents good value for its price, especially when considered as part of the Fritz 17 package. As mentioned in Part I of this review, Fritz 17 includes Fat Fritz, the traditional Fritz 17 alpha-beta engine (Ginkgo, formerly private), the Fritz 17 GUI or graphical interface, and a six month Premium membership at Playchess.com and Chessbase.com. It also automatically installs Leela, which may be difficult for non tech-saavy users. While there are useful improvements to the GUI – the addition of an opening repertoire training function feels like a direct response to Chessable’s newfound popularity – it’s really Fat Fritz that “sells” Fritz 17. And it’s on that basis that purchasing decisions will undoubtedly be made. Could you get by with Leela and Stockfish, or just Stockfish, or an old version of Fritz 13 for that matter? Of course. But neural networks are the next big thing in computer chess, and with its impressive strength and interesting style, Fat Fritz is at the forefront of where chess is heading. Analysts, enthusiasts, and improvers will want to have it as part of their toolbox.Quick links: Fritz 17 at US Chess Sales Getting started with Leela Leela UCI options (applicable to Fat Fritz as well) CEGT Rating 40/20 List SPCC NN Rating List All Fat Fritz test games in pgn format 1046 Fat Fritz games, unannotated in pgn Fat Fritz test positions in pgn format All Fat Fritz stories on Chessbase.com
Categories
Archives
- February 2025 (13)
- January 2025 (24)
- December 2024 (34)
- November 2024 (18)
- October 2024 (35)
- September 2024 (23)
- August 2024 (27)
- July 2024 (44)
- June 2024 (27)
- May 2024 (31)
- April 2024 (51)
- March 2024 (34)
- February 2024 (25)
- January 2024 (26)
- December 2023 (29)
- November 2023 (26)
- October 2023 (37)
- September 2023 (27)
- August 2023 (37)
- July 2023 (47)
- June 2023 (33)
- May 2023 (37)
- April 2023 (45)
- March 2023 (37)
- February 2023 (28)
- January 2023 (31)
- December 2022 (23)
- November 2022 (32)
- October 2022 (31)
- September 2022 (19)
- August 2022 (39)
- July 2022 (32)
- June 2022 (35)
- May 2022 (21)
- April 2022 (31)
- March 2022 (33)
- February 2022 (21)
- January 2022 (27)
- December 2021 (36)
- November 2021 (34)
- October 2021 (25)
- September 2021 (25)
- August 2021 (41)
- July 2021 (36)
- June 2021 (29)
- May 2021 (29)
- April 2021 (31)
- March 2021 (33)
- February 2021 (28)
- January 2021 (29)
- December 2020 (38)
- November 2020 (40)
- October 2020 (41)
- September 2020 (35)
- August 2020 (38)
- July 2020 (36)
- June 2020 (46)
- May 2020 (42)
- April 2020 (37)
- March 2020 (60)
- February 2020 (38)
- January 2020 (45)
- December 2019 (35)
- November 2019 (35)
- October 2019 (42)
- September 2019 (45)
- August 2019 (56)
- July 2019 (44)
- June 2019 (35)
- May 2019 (40)
- April 2019 (48)
- March 2019 (61)
- February 2019 (39)
- January 2019 (30)
- December 2018 (29)
- November 2018 (51)
- October 2018 (45)
- September 2018 (29)
- August 2018 (49)
- July 2018 (35)
- June 2018 (31)
- May 2018 (39)
- April 2018 (31)
- March 2018 (26)
- February 2018 (33)
- January 2018 (30)
- December 2017 (26)
- November 2017 (24)
- October 2017 (30)
- September 2017 (30)
- August 2017 (31)
- July 2017 (28)
- June 2017 (32)
- May 2017 (26)
- April 2017 (37)
- March 2017 (28)
- February 2017 (30)
- January 2017 (27)
- December 2016 (29)
- November 2016 (24)
- October 2016 (32)
- September 2016 (31)
- August 2016 (27)
- July 2016 (24)
- June 2016 (26)
- May 2016 (19)
- April 2016 (30)
- March 2016 (36)
- February 2016 (28)
- January 2016 (32)
- December 2015 (26)
- November 2015 (23)
- October 2015 (16)
- September 2015 (28)
- August 2015 (28)
- July 2015 (6)
- June 2015 (1)
- May 2015 (2)
- April 2015 (1)
- February 2015 (3)
- January 2015 (1)
- December 2014 (1)
- July 2010 (1)
- October 1991 (1)
- August 1989 (1)
- January 1988 (1)
- December 1983 (1)