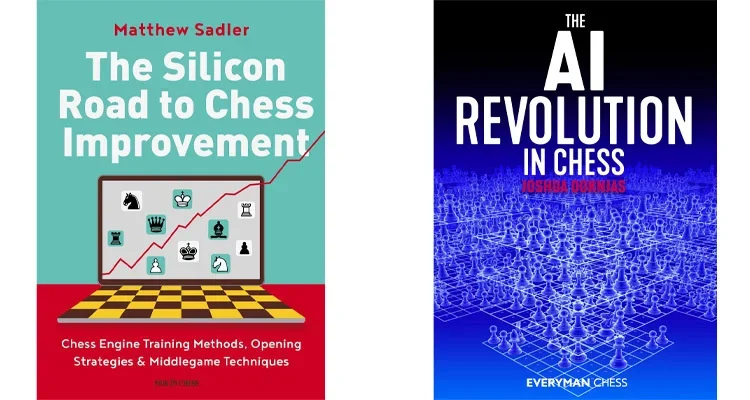
The world of chess engines took a dramatic turn in 2017 when AlphaZero, with just a few hours of self-training, became the strongest chess playing entity on the planet. Soon Game Changer, a 2018 book about AlphaZero by GM Matthew Sadler and WIM Natasha Regan, was an award-winning bestseller. And in time AlphaZero’s self-evaluating AI approach was incorporated into other engines, resulting in continuous advances in strength to this day.
In the Epilogue to Game Changer, the authors state:
Traditional chess engines like Stockfish or Houdini are tremendously strong in unravelling complex tactics in a fraction of the time it would take without their help.
However it is rare to be able to distil general lessons about chess from their calculation-heavy style of play. In contrast, the sense of purpose and direction in the way AlphaZero plays is palpable. This explains why AlphaZero’s games are such a fertile and intuitive learning ground for human chess players.
Sadler’s latest work, The Silicon Road to Chess Improvement, promises to expand upon that last thought by showing how humans can improve their games and understanding by using the latest chess engines. He begins with a very useful chapter on the basics of engine searches and evaluation (particularly the self-learned evaluation of the neural network engines), and the nature and strengths of top engines like AlphaZero, Stockfish Classical, Stockfish NNUE, Leela Zero, AllieStein, Stoofvlees, Komodo, and a few others.
To grossly oversimplify, the older-style engines (like Stockfish Classical, with what he calls ‘hand-crafted evaluations’) have continuously improved and are generally superior in calculating outrageously deep and ingenious tactics. Nevertheless, the neural network engines like Leela Zero with self-learnt evaluations can play a more profound and effective strategic (‘positional’) game, which tends to outperform the calculating monsters. While all of these engines are (literally) superhuman in strength, Stockfish 15, a hybrid engine incorporating a neural network, is currently the strongest of the lot.
Arguably the most relevant chapter in The Silicon Road for those looking to improve their game is called “Training with Engines.” Sadler lays out seven ways of using engines with explanations and detailed examples:
- Training approach #1: Playing rapid games (for example 15 minutes + 10 seconds increment) against Stockfish
- Training approach #2: Playing against Leela Zero restricted to a one-node search.
- Training approach #3: Playing out positions against an engine at a rapid time control (for example 15 minutes + 10 seconds increment)
- Training approach #4: Playing ‘correspondence chess’ against your engine
- Training approach #5: Running engine matches from key opening positions and summarizing the results
- Training approach #6: Letting your engines analyze an opening position for x hours (deep analysis)
- Training approach #7: Periodic checking of your analysis with a live engine
The titles are self-explanatory, and he gives examples of his own training games in each mode. I feel that all these approaches will be useful, but I can’t judge whether they are superior to human online play or other modes of study, or even whether one would gain any particular insight into the way the engine makes those superior evaluations. (More on this concern later.)
For me, training approach #2 is particularly interesting, because in some sense, it separates ‘strategy’ from calculation. Remarkably, Leela at a one-node search depth (using pure evaluation to choose a move, with no calculation) was able to score over 20% against GM Sadler in a 101-game rapid play match... despite not calculating!
If you could find a good way to describe what ‘ideas’ lie behind the neural network engine’s ability to positionally outplay the hand-crafted engines (which it does regularly), you’d think those qualities might stand out more clearly in this one-node situation. Therein lies the rub: trying to describe in words how a human would have to think to ‘understand’ what Stockfish NNUE ‘knows’ about a position that Stockfish Classical doesn’t may be an impossible task. If you go over Sadler’s astonishingly detailed notes to engine games, you see that such brilliant evaluation depends upon many tiny details, the alteration of any of which would change the assessment. The general guidelines and tips he gives cannot encompass such niceties.
This leads us to the general question of whether engines provide a promising path to improvement. It’s not clear to me that Sadler himself feels that he has actually become stronger (in terms of being able to achieve better results) by training with engines, or by absorbing general strategic lessons from them. Nor, to be fair, does he claim this. But he obviously appreciates the brilliance of engine play, and he says that certain factors of modern engine play have become more important for him to consider than he previously understood.
Among these notable factors, he mentions: piece mobility, whole board play, attacking on different wings, rook-pawn advances, slow moves, and many methods of play such as ‘exchanging active pieces to leave the opponent with passive ones,’ ‘developing major pieces by giving away the pawn(s) in front of them,’ and ‘invading through the channel the opponent opened.’ These are instructive sections of the book, and easy to relate to.
On a practical level, Sadler notes that he is (after writing The Silicon Road) more aware of the factors listed above, and he believes he has a better feel for maneuvers that enhance their effectiveness. For all of this, I can’t help but suspect that incorporating these feelings into making actual moves is another matter, possibly only achievable by players with an already sophisticated chess understanding.
Here’s a typical example from the chapter ‘Queen versus pieces,’ which typifies the depth and precision of modern opening preparation with engine assistance:
One of Sadler’s particular interests is in using modern engines to examine key opening tabiya, and many examples in the book turn into painstaking opening investigations. Since these openings tend to have some theory that extends into the endgame, a whole gamut of position types gets tested.
If you’d like to know how to handle both sides of the above position after 1. d4 Nf6 2. c4 g6 3. Nc3 Bg7 4. e4 d6 5. Nf3 0-0 6. Be2 e5 7. 0-0 Nbd7 8. Qc2 c6 9. d5, for example, a single Leela Zero – Stockfish NNUE game includes 16 embedded engine games analyzing separate alternatives just through move 15! Sadler adds considerable commentary trying to explain in words what’s going on. And, curiously, after 39 moves of astonishingly complex play involving both positional and tactical piece sacrifices, Sadler mentions that a different engine game between Stockfish NNUE and Komodo reached exactly the same position, and ended with a wild sequence leading to perpetual check! Obviously the reader isn’t supposed to memorize the game from start to finish, but this kind of investigation is very much along the lines of how the world’s elite players are preparing these days.
Another book which investigates the nature and impact of neural network engines is FM Joshua Doknjas’ The AI Revolution in Chess. Like Sadler, Doknjas organizes his book around well-annotated games, but he doesn’t set up engine-versus-engine matches. Rather he annotates recent games (mainly human-versus-human, between super GMs), using both neural network (AI) and classical engines to explain how the new engines have influenced the way the game is being played today.
Doknjas emphasizes opening theory even more than Sadler, and his first four chapters (199 pages of the book) are detailed examinations of the Grünfeld (“arguably the opening that has been impacted the most by modern engines”), the Catalan, the Najdorf, and a few ‘AI-inspired’ openings like 1. d4 Nf6 2. c4 g6 3. h4.
This last example is particularly interesting. Modern games with the Grünfeld have featured (h2-)h4-h5-h6 repeatedly at even the top levels, particularly in the Exchange Variation line 1. d4 Nf6 2. c4 g6 3. Nc3 d5 4. cxd5 Nxd5 5. e4 Nxc3 6. bxc3 Bg7 7. Bc4 (but also with 7. Nf3); after both sides develop in standard fashion over the next six to eight moves, White plays h2-h4 more-or-less out of the blue.
The advance of this pawn to h6 (which is arguably the main difference between the engine treatment and previous grandmaster practice) is to either shut in or suffocate Black’s dark-squared bishop and/or to take away squares from the king and create tactical opportunities. It’s interesting that the same engines that influenced White towards the h4-h5-h6 scheme have provided Black with effective counter-strategies. From both Doknjas’ analysis and recent over-the-board and correspondence games, we see that White’s ideas that initially led to success are running into effective defenses.
In the Catalan, White has tried a wide variety of pawn sacrifices, some of which have a kind of long-term compensation that engines are so much better at seeing than humans. Anyone who has followed Catalan theory over the years will recognize how much this staid old opening has been spiced up and expanded by dynamic engine ideas.
In discussing the Najdorf, Doknjas notes that “the older engines struggle against the newer ones in closed, strategic middlegames because concrete calculation is less effective in such positions,” and that “the newer engines have the remarkable ability to bring long-term strategic ideas into the sharpest positions.” From the examples — even in highly tactical lines — the AI engine will very often beat a classical engine which is superior in calculation.
The rest of the book deals mainly with middlegame ideas such as pawn sacrifices, closed positions, and material imbalances. As with similar sections of Sadler’s work, the examples are excellent but it’s not clear that a human player can translate the strategic generalities into practical success. Doknjas continues to use games with highly theoretical contemporary openings to make his points, which is a nice bonus for students.
As someone who keeps up with French Defense theory (I write a monthly column with the latest games for ChessPublishing.com), I was curious whether these monsters had any breakthroughs which might advance theory or introduce novel strategies. Sadler’s book has a chapter called “French Structures,” and has nine games with the French Defense.
In contrast to other openings he deals with, there are few, if any, games in important theoretical lines, and we don’t get to see how a super-engine might handle any of the currently successful variations for Black. I didn’t feel I learned much about the French from Sadler, although I did find one fine example instructive and enlightening:
Doknjas has some more theoretically relevant examples in the French, including this one:
Both of these books are full of wonderful games with stunning strategies that seem to come from another planet. This in itself makes them enjoyable and rewarding. Both authors’ annotations are instructive but also show an enthusiastic appreciation of the engines’ ingenuity.
On a more philosophic level, the alien nature of computer play inevitably raises difficult questions about whether engines can be reasonably said to have ‘ideas’ or ‘intuitions.’ More importantly, it remains to be shown whether and how humans can utilize and benefit from such ideas. Sadler answers this latter question with an enthusiastic ‘yes,’ and Doknjas is optimistic if more cautious.
I have to admit that the most impressive examples of strategic thinking in these books seem indescribable to me and out of the grasp of human reasoning. The moves simply work because dozens of minor factors conspire together. For example, an engine sacrifices material for extremely long-term compensation because it can work out the consequences of various counter-sacrifices or attempts at simplification. A human, subject to calculational imperfection, would imagine those potential pitfalls stretching well ahead into the future and conclude that the probability of success is too low to make the sacrifice practical. What the engine is doing not only can’t be put into words, but is essentially too complex to be understood by us.
So yes, these books convince me that engine study can lead to improvement, but generally in fairly narrow and specific contexts. First, by finding exact orders, well-timed maneuvers, and successful plans in the opening, as is practiced by every leading player in the world. More generally, in discovering typical maneuvers in certain structures and better evaluation of contrasting strategies — for example, certain pawn sacrifices or flank attacks.
Once you move beyond this sort of specific knowledge, however, I think it becomes fruitless to try to ‘think like an engine.’ Sometimes you may well have no idea why the engine is pointing you in a certain direction, at which point using your own chess judgment can be a good way to get back to the real world.
I would argue that a more modest but meaningful way to benefit from studying engine games — and one endorsed by both authors — would be to refine your sense of the factors that an engine seems to value in particular kinds of positions, for example, mobility, space, or centralization. In that sense, studying with engines isn’t so different from studying games collections or even your own games. The nice part is that you have a genius by your side to keep your mind open and surprise you with new ideas.
Doknjas, Joshua. The AI Revolution in Chess. Everyman Chess, 2002. ISBN 9781781945988, 336 pages. (Available from uscfsales.com, $28.95.)
Sadler, Matthew. The Silicon Road to Chess Improvement. New in Chess, 2022. ISBN 9789056919832, 496 pages. (Available from uscfsales.com, $29.95.)
Categories
Archives
- April 2024 (44)
- March 2024 (34)
- February 2024 (25)
- January 2024 (26)
- December 2023 (29)
- November 2023 (26)
- October 2023 (38)
- September 2023 (27)
- August 2023 (37)
- July 2023 (47)
- June 2023 (33)
- May 2023 (37)
- April 2023 (45)
- March 2023 (37)
- February 2023 (28)
- January 2023 (31)
- December 2022 (23)
- November 2022 (32)
- October 2022 (31)
- September 2022 (19)
- August 2022 (39)
- July 2022 (32)
- June 2022 (35)
- May 2022 (21)
- April 2022 (31)
- March 2022 (33)
- February 2022 (21)
- January 2022 (27)
- December 2021 (36)
- November 2021 (34)
- October 2021 (25)
- September 2021 (25)
- August 2021 (41)
- July 2021 (36)
- June 2021 (29)
- May 2021 (29)
- April 2021 (31)
- March 2021 (33)
- February 2021 (28)
- January 2021 (29)
- December 2020 (38)
- November 2020 (40)
- October 2020 (41)
- September 2020 (35)
- August 2020 (38)
- July 2020 (36)
- June 2020 (46)
- May 2020 (42)
- April 2020 (37)
- March 2020 (60)
- February 2020 (39)
- January 2020 (45)
- December 2019 (36)
- November 2019 (35)
- October 2019 (42)
- September 2019 (45)
- August 2019 (56)
- July 2019 (44)
- June 2019 (35)
- May 2019 (40)
- April 2019 (48)
- March 2019 (61)
- February 2019 (39)
- January 2019 (30)
- December 2018 (29)
- November 2018 (51)
- October 2018 (45)
- September 2018 (29)
- August 2018 (49)
- July 2018 (35)
- June 2018 (31)
- May 2018 (39)
- April 2018 (31)
- March 2018 (26)
- February 2018 (33)
- January 2018 (30)
- December 2017 (26)
- November 2017 (24)
- October 2017 (30)
- September 2017 (30)
- August 2017 (32)
- July 2017 (27)
- June 2017 (32)
- May 2017 (26)
- April 2017 (37)
- March 2017 (28)
- February 2017 (30)
- January 2017 (27)
- December 2016 (29)
- November 2016 (24)
- October 2016 (32)
- September 2016 (31)
- August 2016 (27)
- July 2016 (24)
- June 2016 (26)
- May 2016 (19)
- April 2016 (30)
- March 2016 (37)
- February 2016 (27)
- January 2016 (33)
- December 2015 (25)
- November 2015 (23)
- October 2015 (16)
- September 2015 (28)
- August 2015 (28)
- July 2015 (6)
- June 2015 (1)
- May 2015 (2)
- April 2015 (1)
- February 2015 (3)
- January 2015 (1)
- December 2014 (1)
- July 2010 (1)
- October 1991 (1)
- August 1989 (1)
- January 1988 (1)
- December 1983 (1)